Recent technological advancements in genomics and imaging have resulted in a vast increase in molecular and cellular profiling data, presenting challenges for traditional analysis methods. Modern machine learning, particularly deep learning, offers solutions by handling large datasets to uncover hidden structures and make accurate predictions. This article explores deep learning applications in regulatory genomics and cellular imaging, detailing how these techniques work when they are most effective and potential challenges. Deep learning, a subset of machine learning, automates the critical step of feature extraction, improving the performance of predictive models without requiring predefined assumptions about underlying mechanisms. Deep learning captures complex functions by transforming raw data into abstract feature representations through multiple neural network layers. It has shown significant advancements in image and computational biology.
Machine learning methods appeal to computational biology because they build predictive models without knowledge of biological mechanisms. For example, predicting gene expression levels from epigenetic features or the viability of cancer cell lines exposed to drugs involves training models like support vector machines or random forests. Though sometimes seen as black boxes, these models offer valuable predictions even if the underlying biological interactions remain unclear. The review emphasizes the importance of data preprocessing, feature extraction, model fitting, and evaluation in the machine learning workflow. It highlights the shift from manual to automated feature extraction through deep learning. It provides practical guidance for applying these techniques in biology, discussing current software, potential pitfalls, and how deep learning compares to traditional methods.
Deep Learning Transformations in Regulatory Genomics:
Traditional methods in regulatory genomics map sequence variation to molecular traits by identifying regulatory variants that affect gene expression, DNA methylation, histone marks, and proteome variation. However, these methods have limitations, as the variation in the training population constrains them and requires large sample sizes to study rare mutations. Deep neural networks offer advantages by learning features directly from sequence data and capturing nonlinear dependencies and interactions across wider genomic contexts. They have been effectively used to predict splicing activity, DNA- and RNA-binding protein specificities, and epigenetic marks, demonstrating their potential in understanding DNA sequence alterations.
Early Applications and Advances of Neural Networks in Regulatory Genomics:
Initial applications of neural networks in regulatory genomics enhanced classical methods by using deep models without altering input features. For example, a fully connected feedforward neural network predicted splicing activity using pre-defined features, achieving higher accuracy and identifying rare mutations. More recent advances employ CNNs to train directly on DNA sequences, eliminating the need for pre-defined features. CNNs reduce model parameters by applying convolutional operations to small input regions and sharing parameters, allowing for effective prediction of DNA- and RNA-binding protein specificities and functional single nucleotide variants.
Advances in Predicting Mutation Effects and Joint Trait Predictions Using Deep Learning:
Deep neural networks applied to raw DNA sequences can predict the effects of mutations in silico, complementing QTL mapping and aiding in identifying rare regulatory SNVs. Mutation maps visually represent these effects. Advances in CNNs allow predicting multiple traits, such as chromatin marks and DNase I hypersensitivity, from larger DNA sequence windows. Multitask learning and CNN-based models, like Basset, have improved performance and computational efficiency. Additionally, RNNs and unsupervised learning models offer alternative feature extraction and classification methods in regulatory genomics.
Deep Learning in Biological Image Analysis:
Deep neural networks, particularly CNNs, have significantly advanced biological image analysis. Early applications focused on pixel-level classification, such as predicting cell structures in C. elegans embryos and detecting mitosis in breast histology images. These models outperform traditional methods like Markov random fields. Innovations like U-Net improved localization by integrating fine-grained information from early layers. Beyond pixel-level tasks, CNNs classify whole cells, tissues, and even bacterial colonies, outperforming handcrafted feature methods. The trend is towards end-to-end analysis pipelines utilizing large bioimage datasets and the powerful symbolic capabilities of CNNs.
Conclusion:
Deep learning methods enhance traditional machine learning tools and analysis strategies in computational biology, including regulatory genomics and image analysis. Early software frameworks have simplified model development and provided accessible tools for practitioners. Ongoing improvements in software infrastructure are expected to broaden the application of deep learning to more biological problems.
Sources:
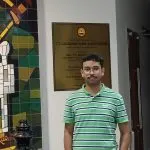
Sana Hassan, a consulting intern at Marktechpost and dual-degree student at IIT Madras, is passionate about applying technology and AI to address real-world challenges. With a keen interest in solving practical problems, he brings a fresh perspective to the intersection of AI and real-life solutions.