The research field concerned with this study revolves around advancing machine reasoning capabilities. This field explores the intersection of language, agent, and world models, focusing on enhancing AI systems’ reasoning and planning abilities. This interdisciplinary field draws upon cognitive science, linguistics, computer science, and artificial intelligence to develop more robust and versatile reasoning mechanisms for machines, especially in complex real-world scenarios.
The primary problem addressed in this research is the inherent limitations in current LLMs regarding consistent reasoning and planning across diverse scenarios. These limitations include the ambiguity and imprecision of natural language, the inefficiency of language as a medium for reasoning in certain situations, and the need for real-world grounding and context. The research aims to overcome these challenges by introducing a more integrated and comprehensive framework for machine reasoning.
Presently, machine reasoning predominantly relies on LLMs. These models have shown strong capabilities in language tasks but face limitations in inference, learning, and modeling, particularly in real-world and social contexts. The existing approaches need to simulate actions efficiently and their effects on world states, leading to inconsistent reasoning and planning. The research identifies these gaps as critical areas for improvement.
The researchers from UCSD and JHU propose a framework known as the LAW framework, integrating language models, agent models, and world models. This framework aims to enhance the reasoning capabilities of machines by incorporating essential elements of human-like reasoning, such as beliefs, goals, anticipation of consequences, and strategic planning. The LAW framework is a more effective abstraction for machine reasoning, overcoming the limitations of current LLM-based methods.
The LAW framework has shown promising results in structuring LLM reasoning with future state prediction and strategic planning. It addresses the challenges of complex, uncertain state dynamics in real-world reasoning problems. The approach has led to more data-efficient learning, better generalization in unseen scenarios, and enhanced social and physical commonsense reasoning capabilities.
In conclusion, the research presents an innovative approach to machine reasoning, addressing the critical limitations of current LLMs. Integrating language, world, and agent models in the LAW framework signifies a substantial leap towards more human-like reasoning and planning in AI systems. The framework’s emphasis on multimodal understanding, strategic planning, and real-world grounding could be pivotal in advancing AI capabilities and applications.
Check out the Paper. All credit for this research goes to the researchers of this project. Also, don’t forget to join our 35k+ ML SubReddit, 41k+ Facebook Community, Discord Channel, LinkedIn Group, and Email Newsletter, where we share the latest AI research news, cool AI projects, and more.
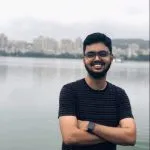
Hello, My name is Adnan Hassan. I am a consulting intern at Marktechpost and soon to be a management trainee at American Express. I am currently pursuing a dual degree at the Indian Institute of Technology, Kharagpur. I am passionate about technology and want to create new products that make a difference.