The relationship between trust and accountability is taking center stage in the global conversations around AI. Accountability and trust are two sides of the same coin.
In a relationship – whether romantic, platonic or business, we trust each other to be honest and considerate. Trust is fueled by actions that showcase accountability. We are more likely to trust individuals who hold themselves accountable for their actions and decisions.
A manager is more willing to trust team members who take personal ownership of projects and complete their tasks on time and to a certain set standard. The team members are likelier to trust a manager accountable for supporting their employees and cultivating a work environment where employees can succeed.
Refusing accountability also harms trust within a relationship. A customer is less likely to trust a bank that previously defaulted on obligations and shifted responsibility. A partner that refuses to explain or justify their risky financial decisions is attempting to skirt accountability and is, therefore, harming the trust in the relationship. However, trust can be fostered by clarity of ownership and responsibility.
The ubiquitous nature of AI raises many questions. Is this a decision a human should be making? Can we trust different AI systems? Who is responsible for them and their actions? How are the outcomes monitored? Who is accountable for the outcomes? Can these outcomes be overridden?
As we talk about trustworthy AI, users, developers and deployers of AI should consider these ways to embed accountability into our AI systems to foster trust.
Assigned accountability for outcomes
Accountability is a shared responsibility of all people and entities that interface with an AI system. Individuals and organizations must recognize the role they play in systems across the lifecycle, from data collection to analytics to decision making. Because of this, individuals should proactively mitigate and remediate adverse impacts of decisions derived from such.
Accountable organizations take the performance and accuracy of their AI systems seriously.
One way to encourage accountability is by building clear decision workflows, which assign ownership and add transparency to the AI system. Decision workflows allow users to create, approve, annotate, deploy and audit decisioning processes while maintaining a trail of who was involved.
This intentional oversight helps build accountability in various decision checkpoints and allows organizations to understand and mitigate risks proactively. Within decision flows, organizations can also build accountability by assigning ownership and responsibility for specific steps of the process and solution outcomes. Clarity on ownership of decision making is essential for operational excellence and team empowerment, which inspires trust in the organization and efforts.
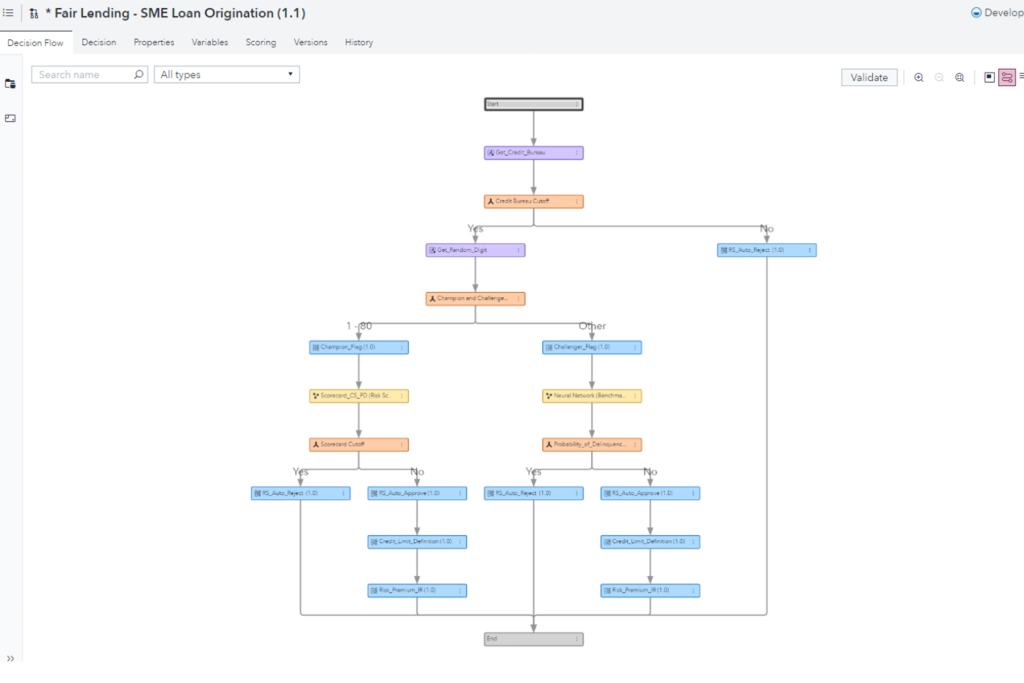
Identify and remediate issues and learn from feedback
Accountability also implies the ability to identify and resolve issues as they arise. An AI system designed with accountability in mind must include mechanisms for customer feedback, error remediation and correction.
Developing and embracing accountability for our models and AI systems can also help foster trust between individual users and society. This creates a robust foundation for responsible AI innovation.
Observing and auditing AI and analytics flows will ensure that the organization is alerted to any issues as soon as possible and can respond swiftly. Quick response to issues will allow the organization to mitigate concerns before they escalate proactively. Implementing feedback loops helps AI systems to learn and adjust their behavior accordingly.
For example, spam filters use user feedback to improve their spam flagging capabilities. Medical diagnosis systems can use feedback from doctors to improve their diagnostic abilities.
Self-driving car systems use feedback from sensors and cameras to improve their ability to navigate the road. More accurate functionality inspires users to trust the system’s capabilities.
Keeping a watchful eye for the unexpected
Accountable organizations take the performance and accuracy of their AI systems seriously. These organizations are intentional in preempting and curtailing the impact of unexpected behaviors as well as granting remediation to impacted parties. They also make efforts to provide remedies to those who may be affected by these behaviors.
To effectively identify and address any adverse impacts in advance, continuous monitoring of AI systems is crucial.
One of the ways to achieve this is by implementing performance monitoring. This involves keeping an eye on various aspects of AI system performance, such as data drifts, concept drifts, out-of-bounds values and other metrics like Lift, ROC, average squared errors, and so on.
These monitoring mechanisms help organizations build the right level of accountability by looping inappropriate entities and measures needed to rectify unexpected behaviors of AI systems.
Fig. 2: Performance monitoring in SAS Model Manager lets users automatically monitor input variable distribution over time to make sure data drift is
Source link