The advent of transformer architectures has marked a significant milestone, particularly in their application to in-context learning. These models can make predictions based solely on the information presented within the input sequence without explicit parameter updates. This ability to adapt and learn from the input context has been pivotal in pushing the boundaries of achievable across various domains, from natural language processing to image recognition.
One of the most pressing challenges in the field has been dealing with inherently noisy or complex data. Previous approaches often need help maintaining accuracy when faced with such variability, underscoring the need for more robust and adaptable methodologies. While several strategies have been developed to address these issues, they typically rely on extensive training on large datasets or depend on pre-defined algorithms, limiting their flexibility and applicability to new or unseen scenarios.
Researchers from Google Research and Duke University propose the realm of linear transformers, a new model class that has demonstrated remarkable capabilities in navigating these challenges. Distinct from their predecessors, linear transformers employ linear self-attention layers, enabling them to perform gradient-based optimization directly during the forward inference step. This innovative approach allows them to adaptively learn from data, even in the presence of varying noise levels, showcasing an unprecedented level of versatility and efficiency.
The innovation of this research demonstrates that linear transformers can go beyond simple adaptation to noise. By engaging in implicit meta-optimization, these models can discover and implement sophisticated optimization strategies that are tailor-made for the specific challenges presented by the training data. This includes incorporating techniques such as momentum and adaptive rescaling based on the noise levels in the data, a feat that has traditionally required manual tuning and intervention.
The findings of this study are groundbreaking, revealing that linear transformers can outperform established baselines in tasks involving noisy data. Through a series of experiments, the researchers have shown that these models can effectively navigate the complexities of linear regression problems, even when the data is corrupted with varying noise levels. This ability to uncover and apply intricate optimization algorithms autonomously represents a significant leap forward in our understanding of in-context learning and the potential of transformer models.
The most compelling aspect of this research is its implications for the future of machine learning. The demonstrated capability of linear transformers to intuitively grasp and implement advanced optimization methods opens up new avenues for developing models that are more adaptable and more efficient in learning from complex data scenarios. This paves the way for a new generation of machine learning models that can dynamically adjust their learning strategies to tackle various challenges, making the prospect of truly versatile and autonomous learning systems a closer reality.
In conclusion, this exploration into the capabilities of linear transformers has unveiled a promising new direction for machine learning research. By showing that these models can internalize and execute complex optimization strategies directly from the data, the study challenges existing paradigms and sets the stage for further future innovations.
Check out the Paper. All credit for this research goes to the researchers of this project. Also, don’t forget to follow us on Twitter and Google News. Join our 38k+ ML SubReddit, 41k+ Facebook Community, Discord Channel, and LinkedIn Group.
If you like our work, you will love our newsletter.
Don’t Forget to join our Telegram Channel
You may also like our FREE AI Courses.
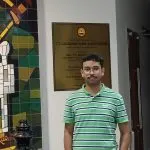
Sana Hassan, a consulting intern at Marktechpost and dual-degree student at IIT Madras, is passionate about applying technology and AI to address real-world challenges. With a keen interest in solving practical problems, he brings a fresh perspective to the intersection of AI and real-life solutions.