Would you like to make your business processes more efficient? Reduce risk while improving the value of services? Stay focused on top priorities and have more time for innovation?
Those are common goals of industries across the globe. And they’re all things you could tag an AI assistant (a digital assistant teammate) to help you accomplish. With their potential to address so many business goals, we predict the use of AI assistants will soar in 2024.
Let’s look at what an AI assistant is, see how it relates to generative AI, and learn three ways organizations could use an AI assistant. All while keeping the human in the driver’s seat.
What is an AI assistant?
Digital assistants, powered by AI technology, can assist people in accomplishing specific objectives. For example, an AI assistant can complete step-by-step tasks, create new tasks and reprioritize task lists. It can follow voice commands, suggest phone calls and answer questions.
Spotting intelligence gaps: An example from law enforcement
Much of the work involved in law enforcement investigations is manual, tedious and procedural. For example, investigators responsible for evaluating witness statements spend countless hours on this crucial but menial task. The enormity of this task multiplies when investigators have to review long, detailed statements from multiple witnesses.
What if an AI-powered virtual assistant could cut down on manual work by reviewing witness statements to identify gaps, suggest ways to fill the gaps, and assist with the follow-up work?
For an example of a simple task, think of a single witness statement with an incomplete vehicle registration. An AI assistant could quickly spot that gap and flag it to the human investigator. Then, the assistant could suggest a next step or task. It could even write an email draft to a CCTV company, asking for video footage that might show the entire license plate number. A detailed record of the whole process would be captured along the way.
To ensure the entire process is handled appropriately:
The investigator would be part of (and in charge of) the process from start to finish.
The thought chain and order of actions taken would be documented in detail.
The AI assistant’s thought chain could be “replayed” at any time to respond to internal or external auditing requirements.
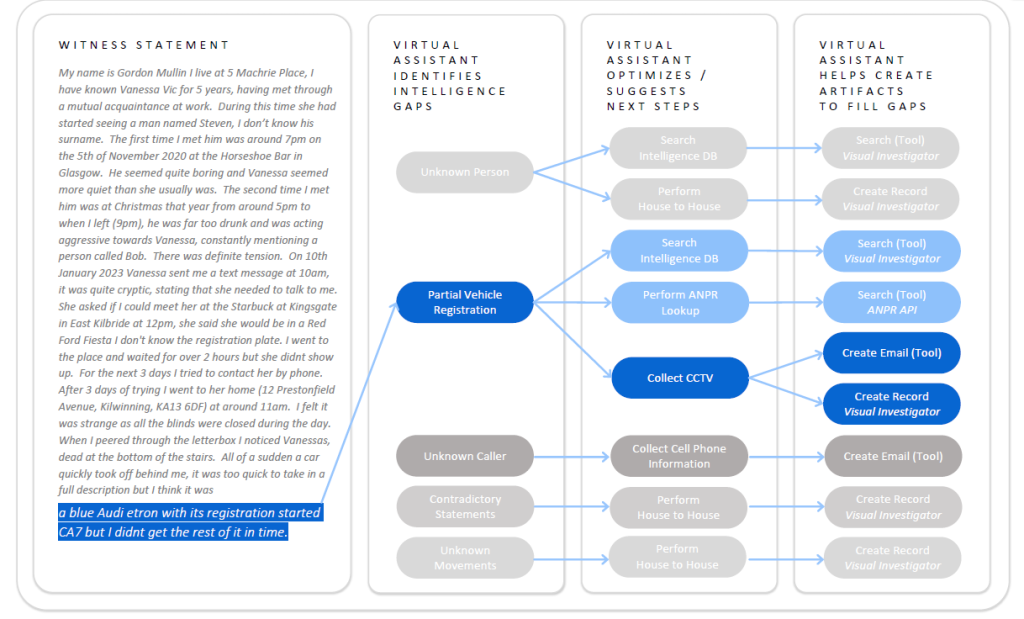
With an AI assistant performing these routine tasks, investigators could stay focused on top priorities – and activities that require their unique insights. In turn, they could accomplish more and potentially catch criminals faster.
This example uses a large language model (LLM), a core generative AI technology based on machine learning. In this case, it’s a conversational AI assistant based on a GPT model (like a chatbot – but more sophisticated).
The same approach would work for other applications, too – for example, anti-money laundering investigations and fraud prevention, health care claim reviews and call center research.
Optimizing yields: A manufacturing scenario
Imagine a plant floor worker at a manufacturing facility who needs to optimize ingredients in the recipe for a wallboard product during a drought. The objective is to get a high yield to avoid wasting ingredients – including water, stucco and additives.
In a typical setting, plant workers use manual trial and error to test and change quantities of different ingredients to improve yield. If they can’t do it independently, they ask the data science or data analytics department to run optimizations for them.
Instead of relying on trial and error or taking time away from other priority work, what if the worker could ask an AI assistant for help?
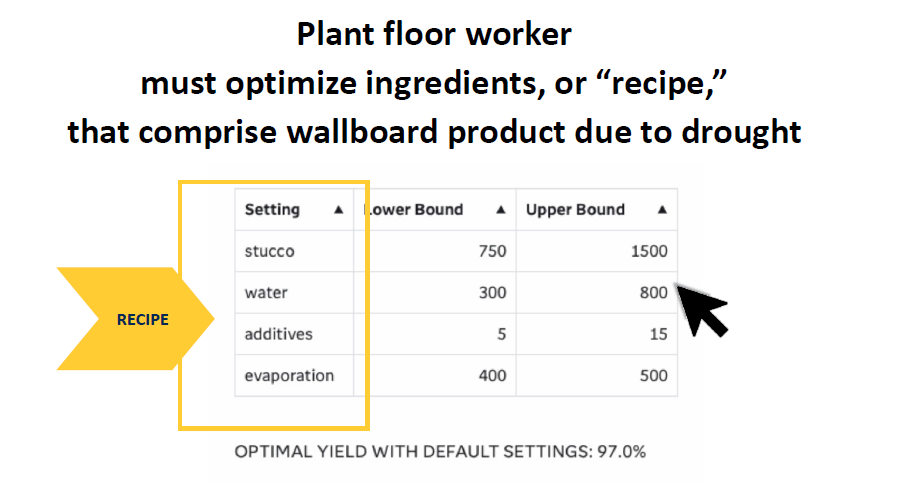
For example, the plant worker could ask a digital assistant to determine which ratios of water, stucco and additives would be best to improve yield in the current state of drought. The AI assistant could quickly evaluate this by changing ratios, such as decreasing upper bounds for water or stucco. Then, it could run optimizations until finding a mixture with an acceptably high yield, such as 96% or higher.
Having a digital teammate would empower plant floor workers to run more optimizations – quickly and independently. No more relying on the IT team for help. No more lost time doing manual tests to find the ideal mixture.
These are just some of the advantages to expect when democratizing analytics across an organization. Flexibility, reduced costs and faster time to value are others.
Jumpstart creativity: A marketing technology example
To engage customers across their life cycles, marketers need to effectively plan engaging campaigns, generate creative content and accurately segment audiences. To achieve great results, these activities require ingenious and time-consuming approaches.
With a trusted digital AI assistant based on an LLM, marketers can:
“Talk” with the assistant through natural language conversations to brainstorm customer audiences and journeys for various marketing strategies.
Develop channel-specific creatives for campaigns.
Generate persuasive, hyper-personalized content for different platforms.
Along the way, the marketer stays in charge of what gets produced, how it’s written or designed, and how it’s used.
Marketers could form highly collaborative relationships with their AI assistants over time. But it’s up to individuals and their organizations to set clear policies and guidelines, use generative AI technology ethically and choose AI assistants that were built with responsible AI at their core.
Learn more about what generative AI means and why it matters